Are baseball arbitration awards fair?
Using unsupervised and supervised learning models to predict salaries
7/23/20242 min read
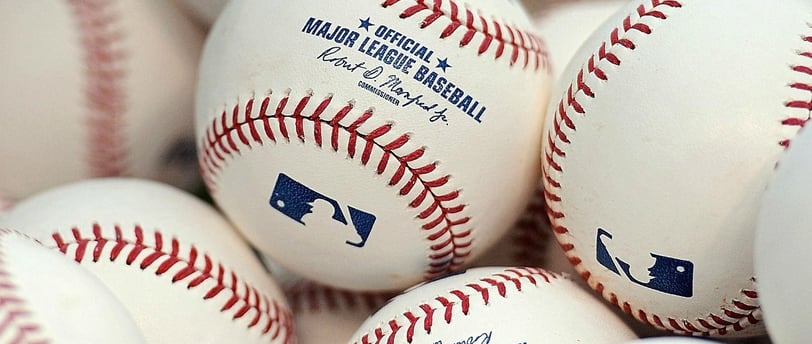
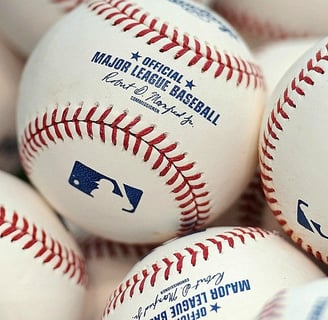
I was deep into my second semester of graduate school, business analytics. April rolled in and I still had not picked a final project for my intermediate statistics course. I had one week remaining to choose a topic and write an abstract, three weeks to complete the analysis, one week to write a paper, and present the results. Gulp. I was following my early morning ritual of drinking coffee and reading the Globe sports section. There was an article on which baseball players won their off-season arbitration cases and which ones lost. Which got me thinking, did the arbitration panel make the correct decision? Were they fair to the players? Let me back up for a minute and explain arbitration for those who do not follow the sport closely.
Professional sports has different rules than other businesses pertaining to acquiring and compensating talent. Professional sports depend on a certain level of parity between teams. Fans need to believe that their team has a chance to win, otherwise they lose interest, and league attendance suffers. One of these rules pertains to when a player can change teams. A baseball player must complete six years of service time before they are free to negotiate a contract with other teams (known as free agency). The players union objected to their salaries being non-negotiable for such a long period of time. Six years could be half of their career. In a compromise with the owners, players were given the right to file for arbitration after their second year of service time. During arbitration, an independent panel would decide if the owners or the player’s proposed salary was most fair. There would be no splitting the difference. One side wins, the other loses.
My hypothesis was that the arbitration awards would be less than the salaries of their peers, when comparing players with similar performance. After all, they the players still lack the leverage of a complete free agent. It turns out I was only partially right. To see what I got wrong you can read the paper I wrote describing the project.
My approach was to use clustering methods to group players with similar offensive statistics. Within each cluster I created the best regression model possible (lowest RMSE) for that group of players and used the regression model to predict the arbitration eligible player ‘s salary. I then compared predicted to actual for each cluster. Clustering does not have labels, so I cannot say the panel was right or wrong. What I could conclude is that the actual versus predicted salaries were much closer together using the clustering method than when I did the same exercise without clustering. The clustering results made more intuitive sense.